Unplanned downtime remains one of the most expensive line items in heavy industry. ABB’s 2023 Value of Reliability survey put the average global cost at ~ USD 125,000 per hour, rising to ~ AUD 349,000 for an Australian plant (with two-thirds of sites losing almost AUD 4.2 M yearly). In parallel, most organisations are locked in a high-stakes race to do more with less: assets are ageing, skilled technicians are retiring, and stakeholder expectations on safety and sustainability are rising.
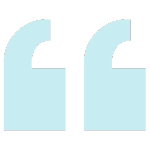
Two-thirds of Australian plants report annual unplanned maintenance costs of more than AUD 4,000,000 per year.
Against this backdrop, Condition-Based Maintenance (CBM) and Predictive Maintenance (PdM) are moving from “promising” to “pragmatic”: leading adopters cut unplanned machine downtime by up to 50% and total maintenance spend by up to 40% while improving forecasting accuracy by as much as 85%, according to Siemens. The shift, however, is not only about sensors and algorithms; it also depends on disciplined, verifiable field execution. Digital inspections provide that missing link.
This article sets out a roadmap, grounded in recent domain data, for achieving data-driven reliability without necessarily over-investing in sensor instrumentation. Digital inspections can fill the sensor gap, acting as a low-cost “human sensor network” that verifies fixes, feeds analytics, and accelerates maturity.
The Five Maintenance Archetypes
Maintenance is the portfolio of planned and unplanned interventions that keep fixed assets safe, available, and efficient across their life cycle.
A solid grasp of each maintenance approach clarifies how businesses can balance cost, risk, and asset longevity. While the terminologies vary slightly across industries, the five most widely recognised strategies are:
Reactive Maintenance (Run-to-Failure)
Under Reactive Maintenance, assets are operated until breakdown. Repairs happen only after failure, often resulting in “firefighting” scenarios. Organisations find this approach appealing in the short term due to minimal upfront planning and lower immediate labour costs. However, it exposes them to unplanned downtime, secondary damage (if a small fault cascades into a major breakdown), and potential safety or environmental hazards. The ABB survey indicates that roughly 20 to 25 percent of industrial firms still rely heavily on run-to-failure, even though unplanned outages can cost hundreds of thousands per hour.
Preventive (Planned) Maintenance
Preventive Maintenance schedules interventions at fixed calendar intervals (e.g., every six months) or usage-based intervals (e.g., every 1,000 production cycles). This method ensures a more orderly approach than Reactive Maintenance. Downtime, though still incurred, becomes at least partially predictable. One major drawback is that many assets do not degrade at a steady rate. Consequently, organisations may invest in unnecessary repairs or replacements. In some cases, over-maintaining equipment can introduce failure modes during reassembly. Despite its shortcomings, Preventive Maintenance remains a common default strategy for many facilities.
Condition-Based Maintenance (CBM)
In CBM, the timing of interventions is driven by objective condition data, such as vibration analysis, thermal imaging, oil particle counts, or ultrasound checks. Once readings exceed predefined thresholds or exhibit trends that suggest imminent problems, an inspection or corrective measure is initiated. The significant advantage is that maintenance actions occur only when needed, saving resources and lowering unplanned failures. Nevertheless, implementing CBM requires investing in condition-monitoring equipment, training staff to interpret data, and integrating these insights into maintenance workflows.
Predictive Maintenance (PdM)
Predictive Maintenance builds on CBM by applying advanced data analytics and often machine learning to forecast exactly when a failure is likely to occur. Rather than purely reacting to threshold breaches, PdM algorithms study historical failure patterns, real-time sensor outputs, and other contextual data (eg, high-quality digital inspection data) to anticipate how an asset’s condition will evolve. The cost of setting up a PdM programme can be significant due to data architecture and analytics expertise, but the payoff is typically high. Studies from Deloitte, McKinsey, and Siemens suggest that well-implemented PdM can reduce unplanned downtime by up to 50 percent and maintenance costs by 40 percent.
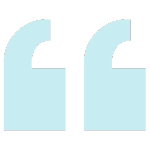
Preventative maintenance can reduce unplanned downtime by up to 50% and maintenance costs by 40%.
Reliability-Centred Maintenance (RCM)
RCM is a structured method that analyses an asset’s functions, its criticality, and potential failure modes to identify the optimal maintenance strategy: reactive, preventive, condition-based, or predictive. RCM demands detailed cross-functional collaboration and in-depth failure mode effects analysis. It aims to ensure that each critical asset is maintained in the most cost-effective way, aligning with safety and business goals. Implementation can be time-intensive, but once finalised, RCM helps prioritise resources efficiently and sets the foundation for stable, long-term reliability.
Strategy | Essence | Typical Use | Pros | Cons |
Reactive | Run-to-failure, fix after breakdown | Low-criticality, low-cost spares | Zero planning cost | Catastrophic downtime; safety risk |
Preventive | Calendar/usage intervals | OEM schedules, safety legislation | Predictable workload | Over-maintenance; hidden failures |
Condition-Based | Thresholds on measured condition | Vibration, oil, thermography | Intervene only when needed | Sensor & interpretation cost |
Predictive | Statistical / ML forecasts of failure | Assets with rich data history | Lowest overall cost; high uptime | Data science and change-management heavy |
Reliability-Centred | Structured mix of the above | Programme optimiser | Systematic, risk-aligned | Resource-intensive upfront |
Where is the industry heading?
The migration is unmistakable: away from time clocks and toward data signals. Sensors, historians, digital inspections, and analytics are converging so that repairs occur just in time, not too late and not too soon.
Milestones for Implementing CBM and PdM
Shifting from time-based or reactive approaches to data-driven programmes demands clear, structured milestones. Based on aggregated insights from major consulting firms (McKinsey, Deloitte) and OEM best-practice guides (Siemens, ABB, Emerson), the following represent key checkpoints:
Identify Asset Criticality and Failure Modes
Begin by ranking assets by their criticality (i.e., how their failure affects production, safety, and costs). Next, identify common failure modes. For instance, rotating equipment might face bearing wear or misalignment, while electrical assets risk overheating or insulation breakdown. This analysis clarifies where to prioritise condition monitoring or advanced analytics.
Data Infrastructure and Sensor Strategy
Review existing data collection methods, sensor coverage, and data flows. Decide which sensors will be installed permanently and which checks will remain manual. In many cases, a partial sensor deployment is most cost-effective, supplemented by digital inspections. Build or upgrade data pipelines so that readings flow seamlessly into historians, analytics platforms, or a centralised maintenance management system.
Condition Indicators and Thresholds
For each high-priority asset, define the condition indicators and thresholds that will trigger action. Examples include a bearing temperature exceeding 85 degrees or a vibration velocity spike beyond the normal envelope. When a threshold is breached, the system should raise an alert and automatically generate a follow-up digital inspection task so technicians can confirm the anomaly, capture evidence and decide whether immediate repair is warranted. Some organisations go further, using machine-learning models that adjust these thresholds dynamically as operating patterns evolve, reducing false alarms while sharpening early-warning accuracy.
Analytics and Predictive Modelling
Once condition data is streaming reliably, adopt more advanced predictive tools. This could be simpler statistical trending or, at a higher level, AI-driven forecasting. Evaluate which software or platform best integrates with your existing systems (e.g., an ERP/CMMS). Pilot predictive models on a small set of assets first.
Operational Integration and Workforce Training
Ensure maintenance teams know how to interpret condition alerts and predictive outputs. Update workflows to support a condition-based or predictive approach: tasks might be triggered automatically in the ERP/CMMS whenever analytics detect anomalies. Provide cross-training or create new roles for reliability engineers who can manage the analytics pipeline.
Pilot Successes and Progressive Scale-Up
After a successful pilot, systematically expand coverage across more assets, lines, or sites. Share pilot achievements with stakeholders to build buy-in. Monitor ROI metrics (e.g., reduced downtime hours, maintenance cost savings, and extended asset life) to reinforce the value proposition.
Continuous Improvement and Prescriptive Evolution
Even after CBM or PdM is widely adopted, refine the approach. Adjust thresholds, add new sensor types, or incorporate advanced algorithms. Some forward-thinking organisations transition to Prescriptive Maintenance. As your data matures, you can embed advanced rules that automatically suggest or schedule interventions, along with recommended steps for technicians (i.e., digital inspection with standardised checklist).
By following these milestones carefully, businesses avoid common pitfalls and enhance the likelihood of a return on their investment. A structured approach also encourages cross-functional alignment. This is particularly vital when dealing with large capital projects, multi-site rollouts, or multi-disciplinary teams.
Sensors: Essential but Not Sufficient
While CBM and PdM promise strong returns, they depend on reliable data from IoT sensors and condition-monitoring devices. OEM reports from Siemens, Schneider Electric, and Emerson highlight that installing sensors throughout an entire plant can be substantial: in certain industrial processes, each installed sensor might cost upwards of 10,000 USD, factoring in hardware, installation labour, connectivity, calibration, and integration with existing systems. Wireless sensor networks are often cheaper than wired systems, but overhead remains considerable.
Given that not every device or subsystem can justify a permanent sensor, bridging strategies become vital. Some organisations opt to equip only critical assets or known failure hotspots with sensors, whilst also integrating advanced digital inspections. Where operators or inspectors use mobile platforms to record and synchronise condition data, photographs, or field measurements, essentially acting as “human sensors”.
Inspectivity – Your Human Sensor Network
Against the backdrop of these developments, Inspectivity positions itself as a digital inspection platform that complements expansive sensor networks where these prove infeasible or prohibitively expensive. While some high-priority assets can justify permanent IoT instrumentation, thousands of other assets across large facilities do not warrant the same investment. Inspectivity helps fill that gap, acting as a structured “human sensor network”.
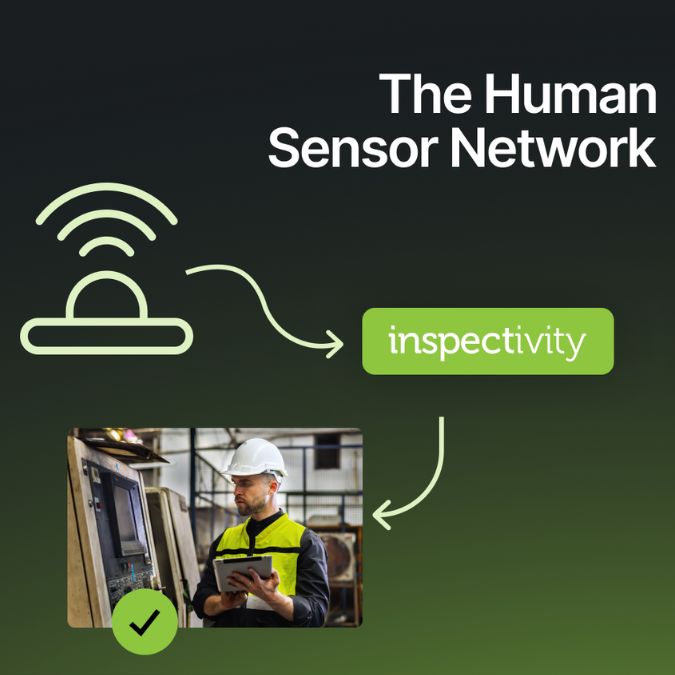
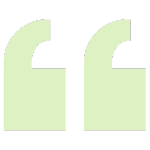
In the age of digital twins and AI-driven reliability, Inspectivity strengthens sensor networks by adding structured, inspectable, and auditable human insights.
Bridging Sensor Gaps
Hardware sensors often focus on easily measurable parameters: vibration, temperature, flow, or pressure. However, many real-world faults are first noticed through visual inspection, subtle noise changes, or the presence of corrosion. Inspectivity Platform ensures that these field observations, previously jotted in paper logs, become digitised, time-stamped, and integrated with the broader maintenance ecosystem. This adds context to sensor readings, providing more holistic asset coverage.
Empowering Condition-Based Programmes
Traditional CBM relies on consistent data inputs. Inspectivity ensures that manual checks are both systematic and standardised. Inspectors follow configurable workflows on a mobile device, capturing strongly typed data fields (like “Surface Temperature: 72 degrees” or “Visible Corrosion: Yes/No”). Such data then becomes part of the condition history, enabling advanced analysis even for assets with no permanent instrumentation.
Laying the Foundation for Predictive Maintenance
By storing digital inspection data in a structured database, Inspectivity helps build the historical dataset that machine learning algorithms require. Over time, repeated inspection records reveal trends that correlate strongly with failures. As an organisation matures, it can integrate Inspectivity’s data with advanced analytics or AI-based PdM solutions. In other words, Inspectivity is not simply a documentation tool; it can be a stepping stone to full predictive maturity.
Integration with EAM and IoT
Inspectivity’s open API approach supports seamless connection to enterprise asset management suites (e.g., SAP PM, IBM Maximo) and IoT sensor data. This integration yields a complete picture of asset status. When a sensor detects an anomaly, a digital inspection can be triggered in Inspectivity. Conversely, if an inspector flags an issue, it can feed a predictive model that refines future predictions. The synergy between human-captured data and machine-captured data increases the accuracy of any CBM or PdM project.
Assuring Compliance and Audit Trails
In many industries, maintenance activities must meet stringent regulatory or insurance requirements. Inspectivity can maintain a secure, traceable record of all checks, photos, and digital signatures. This functionality transforms maintenance from a collection of scattered logs to a thoroughly auditable system that proves the organisation’s adherence to safety, environmental, or quality standards.
Positioning for the Future
As industries move toward prescriptive maintenance, digital twins, and AI-driven reliability engineering, Inspectivity powers this transformation. Even in a scenario with ubiquitous sensors, companies still need a platform that verifies final repairs, documents corrective actions, and captures intangible field observations. By blending digital inspections with sensor data, Inspectivity consistently helps businesses refine their reliability strategies and cut unplanned downtime.
Evidence in Action – Three High-Impact Case Studies
Examining how real organisations apply CBM or PdM reveals tangible results. Here are concise highlights from various industries:
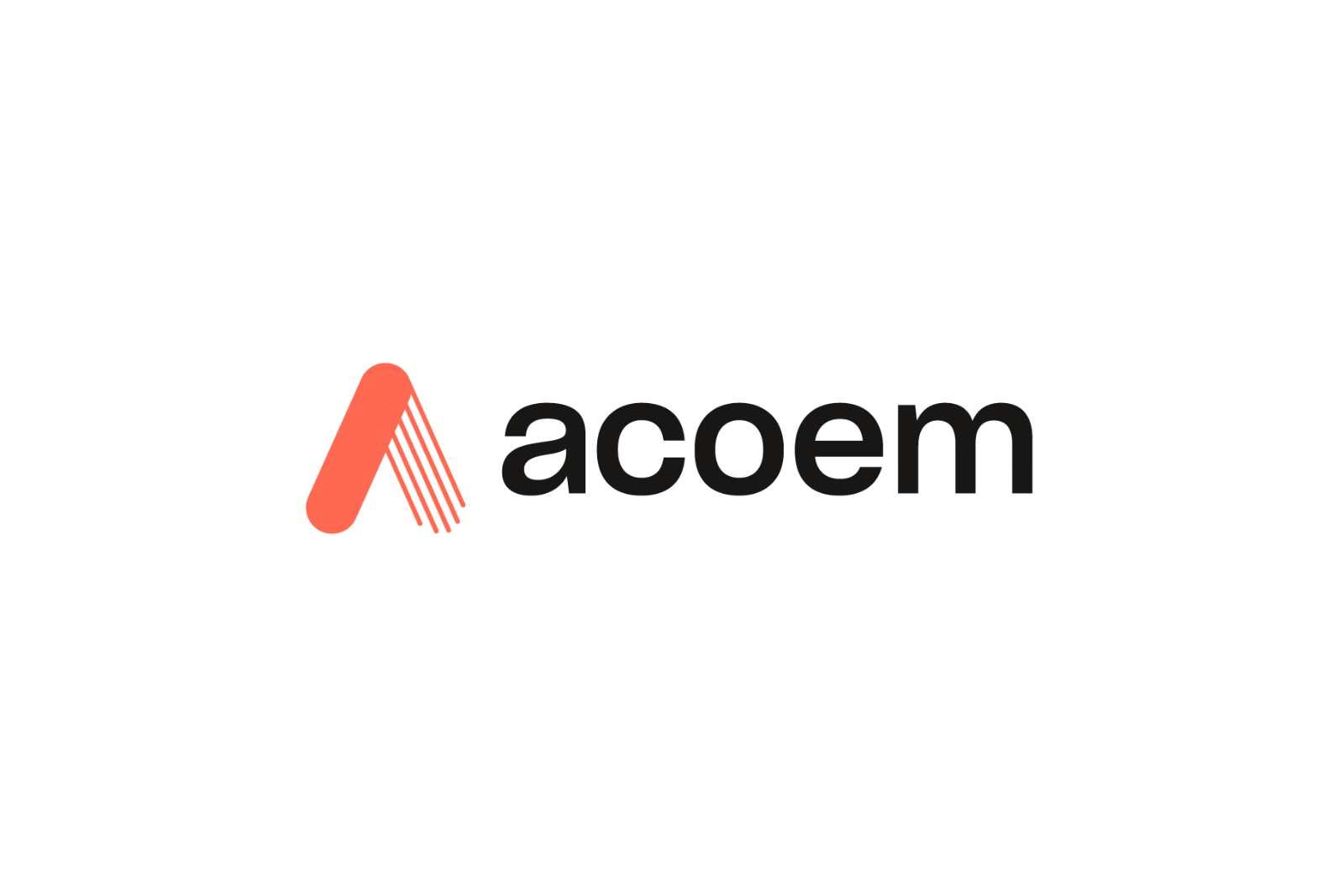
Description: Wireless sensors + Inspectivity-style workflows prevented catastrophic loader failure.
Result: USD 7.8 M cost avoided via early intervention.
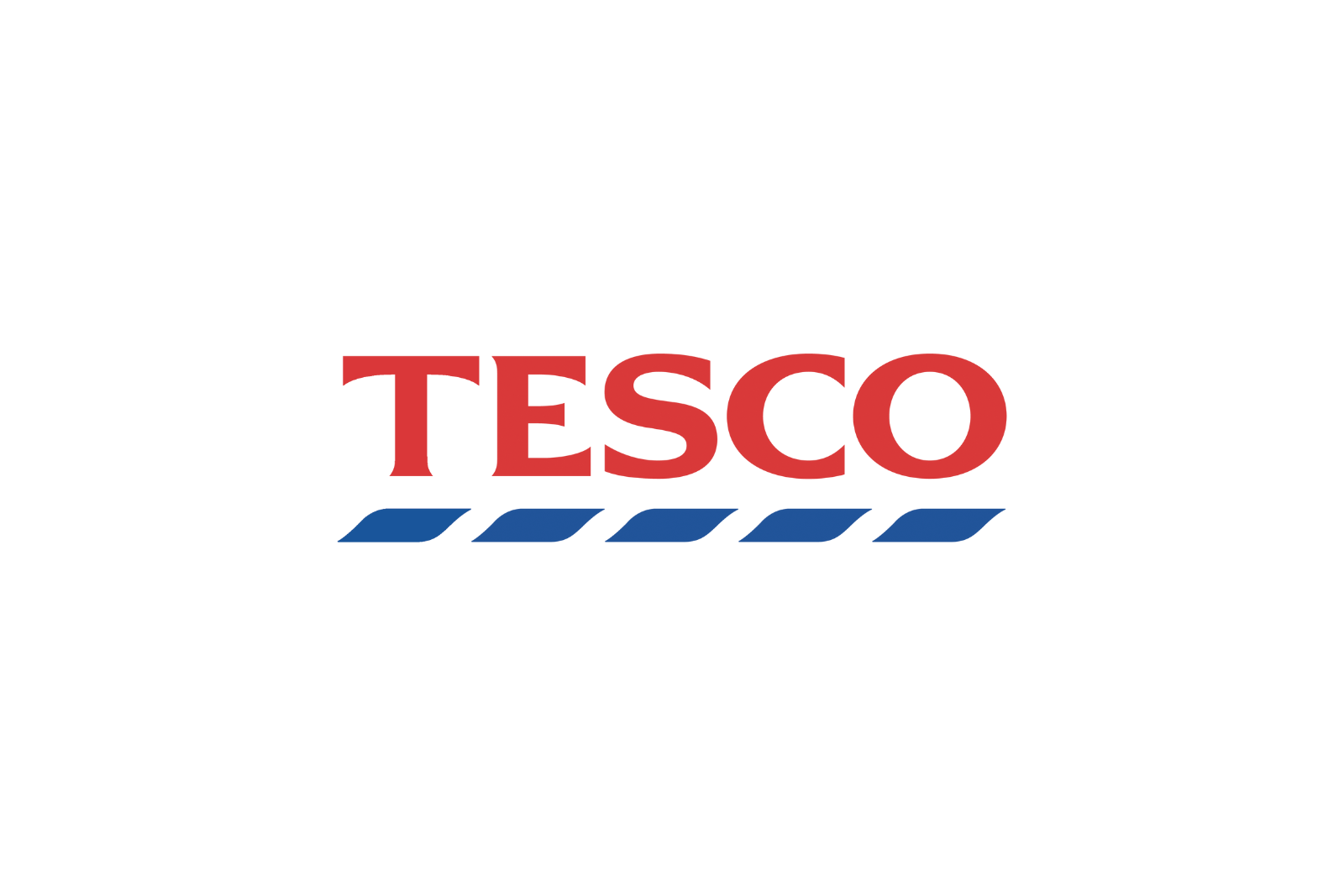
Description: Tablet inspections filled the gap where permanent sensing was impractical; staged journey to CBM.
Result: Instant trend graphs, resulting in earlier fault detection; foundation for PdM.
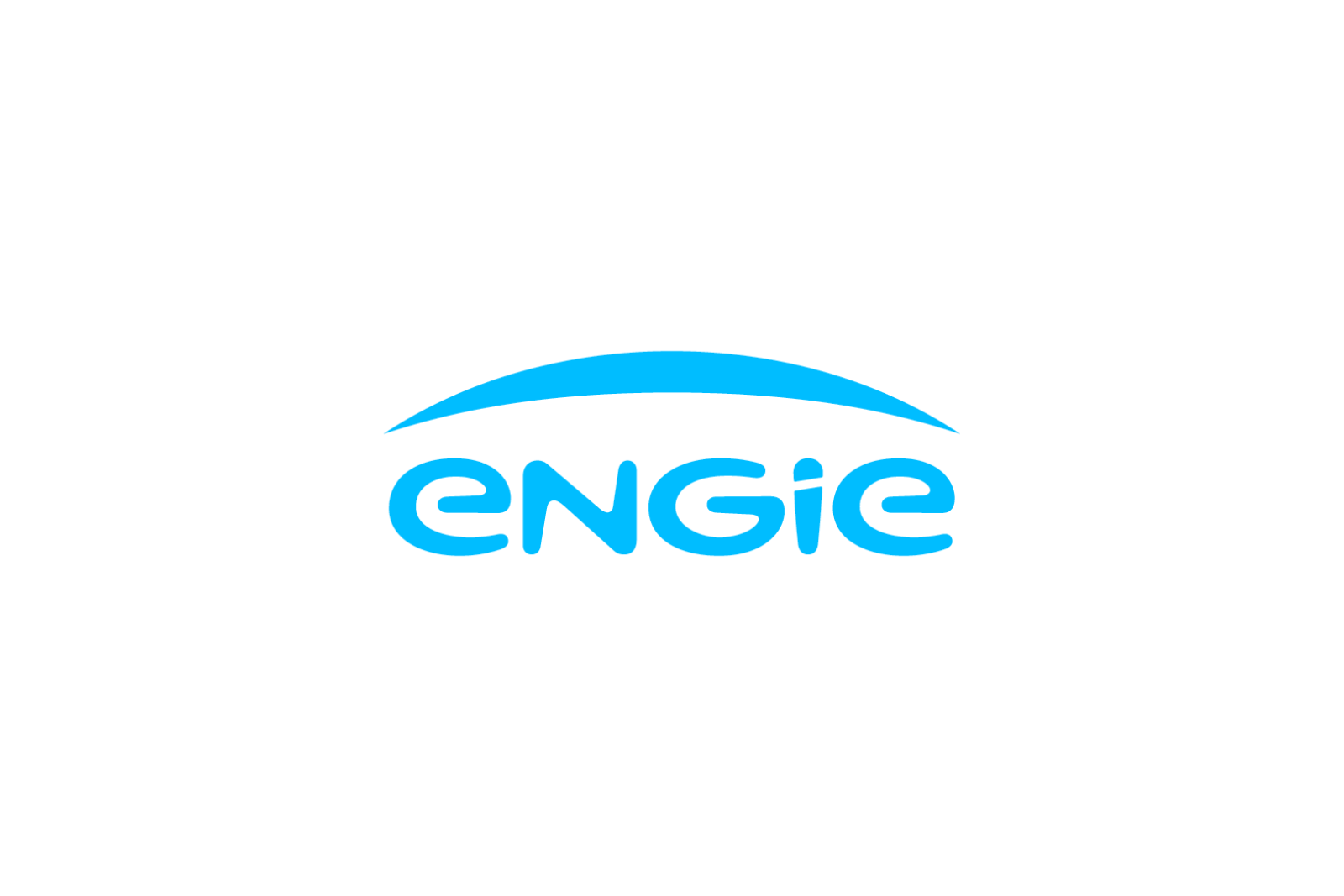
Description: Shows scale when inspection + sensor data feed machine-learning at fleet level.
Result: Up to EUR 800 k annual savings per business unit, 10,000 assets targeted.
Overall, these case studies highlight consistent themes: major savings from minimising reactive repairs, reduced downtime, and improved reliability. Deploying a digital system that merges condition or predictive insights with accessible mobile inspection workflows often unlocks the best outcomes.
Conclusion
Maintenance practices have evolved considerably, from the basic “fix when it breaks” approach to intricate frameworks that continuously learn and predict when interventions are most needed. Condition-Based Maintenance (CBM) and Predictive Maintenance (PdM) stand at the heart of this transformation, driven by ever-improving IoT sensors, big data analytics, and digital platforms that unify field teams with decision-makers. The associated business case is compelling: unplanned downtime can drain millions from corporate accounts, whereas well-executed CBM/PdM strategies substantially reduce these losses, extend asset lifespan, and enhance worker safety.
Downtime still bleeds cash; data-driven maintenance staunches the wound–but only if prediction meets execution. Sensors alert, analytics predict, yet disciplined digital inspections close the last mile between forecast and fix. Inspectivity Platform supplies that discipline: a human-sensor network, an API bridge to your ERP/CMMS and IoT stack, readiness dashboards, and an audit record your CFO and regulator will both trust.
It’s time to move beyond your current maintenance strategy limitations. Ready to modernise your inspection workflows? See why many tier-one operators have decided to make the change. Learn how Inspectivity can help. Book your free trial today.